Published on
In today’s market, we’re inundated with an ever-growing list of both open and closed large language models (LLMs). Well-known offerings like OpenAI’s ChatGPT, Google’s Gemini and Meta’s Llama are proven high performance LLMs. But they are general purpose models that attempt to cover a broad knowledge base but often struggle to produce relevant responses in every domain.
This is why Addepar is building Addison: an LLM ecosystem that will be trained on 15 years of experience serving our clients in wealth management. Addison is not designed to compete with generally available LLMs, but will instead be a closed model that drives solutions where natural language processing can enhance the Addepar experience and our internal processes.
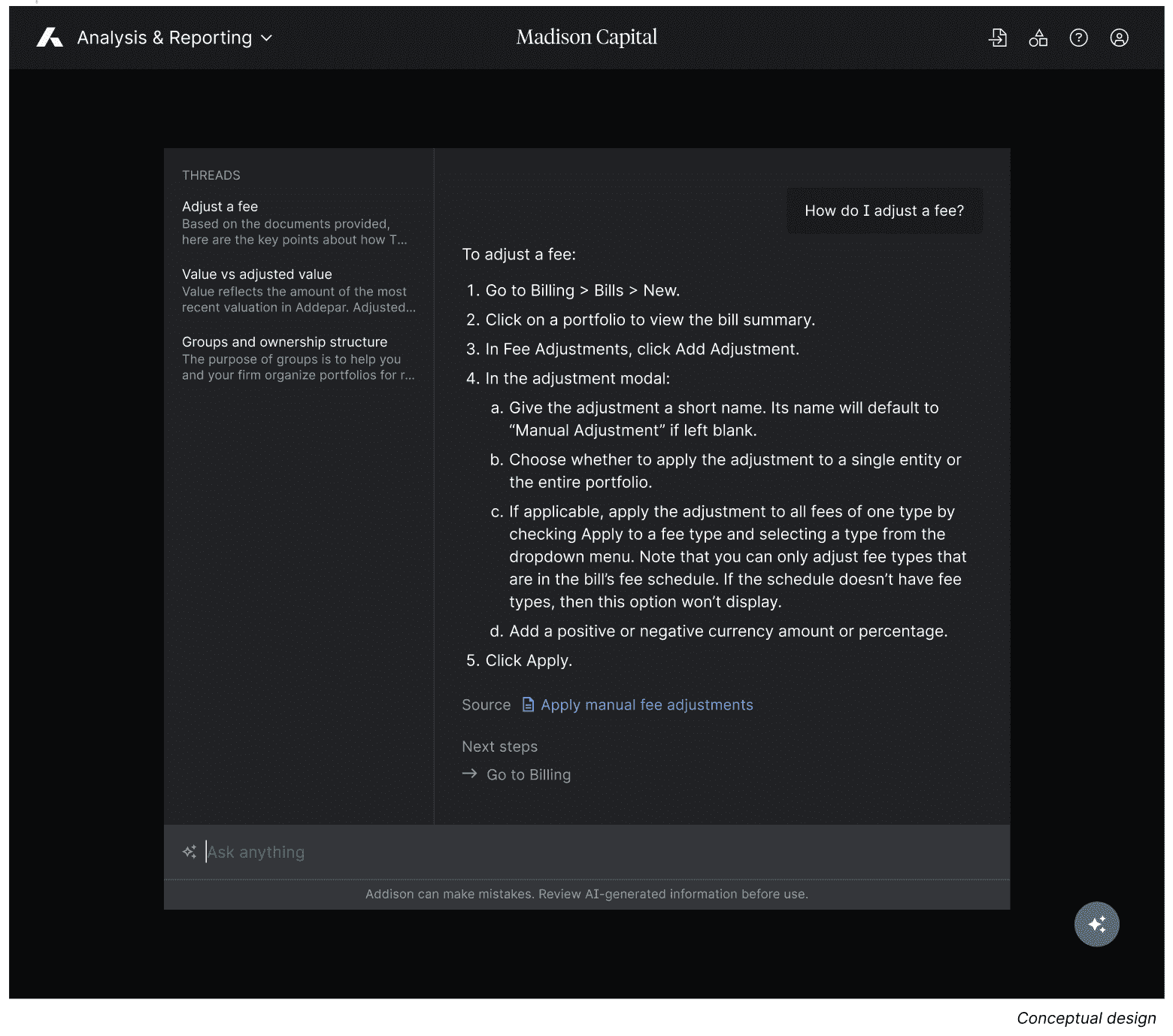
Focused, contextualized knowledge
Addepar has specialized in serving clients in the wealth management industry since 2009. In that time, we’ve accumulated a significant knowledge base of resources that help our clients get the most value out of our platform.
This knowledge base allows us to surpass the accuracy of general-purpose LLMs by addressing questions and tasks specifically contextualized toward Addepar and wealth management workflows. We further enhance this knowledge base with the collective experience of our client support specialists to create the highest quality training dataset for wealth management.
Enhanced security and efficiency
We take the security and privacy of client information very seriously, and protecting the information entrusted to us is our topmost priority. We take full control over Addison’s entire lifecycle, and the training of our custom Addison LLM gives us the exclusive ownership over how it is deployed and used. Training and deploying our own model will allow us to retain full control over Addison’s entire lifecycle, including how the model is constructed and how it is used.
Another important consideration for LLMs is the cost to run inference on the models, where the size of the model is the most important factor in determining this cost. Custom trained models are orders of magnitude smaller than general purpose models, meaning that Addison will be much more efficient– both in terms of computation and how much memory is required.
Powering future AI innovations
Addison will redefine how we interact with data in Addepar. It will power the next generation of Addepar’s user experience and internal automations. Accomplishing all of these use cases efficiently, accurately, and with uncompromised security will transform Addepar and unlock more opportunities for innovation in the future.